Published Date:
Sep 28, 2023
Exclusive
Research
Patient Outcomes
AI
Why Most Published Research Findings Are False
Will future patient outcome models face a rocky future with value-based healthcare
In 2005, Dr. John Ioannidis, a Greek-American physician-scientist, published a groundbreaking essay titled "Why Most Published Research Findings Are False." This thought-provoking piece shed light on the challenges and limitations of scientific research, challenging the prevailing belief that published findings are always reliable. The essay has sparked significant debate and has had a profound impact on the scientific community's understanding of outcome reproducibility and the reliability of scientific studies.
The broader point questions the conventional wisdom that standardized outcome measures are a must for the future of value-based care models.
The complexity of proving a universal standard of measure
Case in point. The Centers for Medicare and Medicaid Services (CMS), in its push for payment model reform suggests that “performance measurement [must] accurately monitor quality and outcomes [by creating] a single set of expectations to reduce provider burden”. But, by that standard it surely raises some astoundingly robotic implications for the future of value-based care.
Which standard, is standard?
This utopia presents a conundrum. It obviously highlights a major conflict for a more personalized medicine. Further, it institutionalizes a rigidity in how our providers might wish to practice in the future – not withstanding how they expect to be compensated for it in an emerging value-based care model.
More importantly, this proliferation of standardized clinical measures now being used in behavioral health may not be proven efficacious in improving the long-term outcome of a patient experiencing a long-term, complex disorder.
There’s a reproducibility crisis in behavioral health measures
Ioannidis's essay addresses a critical issue that underlies the scientific community's credibility—the reproducibility crisis. He argues that a significant number of research findings, even those published in reputable journals, cannot be replicated when attempted by other researchers. This crisis raises questions about the validity of scientific claims and the reliability of research studies that form the foundation of knowledge in various fields.
There’s bias and methodological flaws in standardized standards
Several factors contribute to the high rate of false research findings. One crucial factor is bias, including publication bias, where studies with statistically significant results are more likely to be published, while studies with non-significant or negative results are often disregarded. This bias skews the scientific literature, leading to an overestimation of effect sizes and potentially false claims.
Flawed study designs, small sample sizes, inadequate statistical power, and improper analysis techniques can all contribute to false positive findings. These methodological issues, combined with the pressure to publish novel and groundbreaking results, further exacerbate the problem.
There’s an impact on scientific progress with standardized standards
The implications extend beyond the scientific community. False research findings can have serious consequences, impacting public health, policy decisions, and resource allocation. If policymakers and healthcare professionals base their decisions on unreliable research, it can lead to wasted resources, ineffective interventions, and potential harm to individuals or populations.
All this serves as a wake-up call to the scientific community, researchers, peer reviewers, and journal editors to be vigilant in ensuring research rigor and reproducibility. It emphasizes the importance of transparent reporting, open data sharing, and replication studies to validate research findings and improve the overall quality of scientific research.
Our efforts towards lasting health improvement starts with innovative practices
Since the publication of Ioannidis's essay, the scientific community has made efforts to address the reproducibility crisis. Initiatives like preregistration of studies, replication studies, and increased focus on transparency and data sharing have gained traction. These efforts aim to enhance the reliability and credibility of research, ensuring that published findings are robust and reproducible.
Let’s get real with our view of value
Is there an alternative? Perhaps. Companies like the YOUU Health, with its large cloud-collected data, present alternatives strategies designed for value infrastructure by using real-world data (RWD) versus clinical trial data — two different types of data used in healthcare outcomes and decision-making. Each has its own advantages and disadvantages. Here are some advantages of real-world data compared to standardized outcomes data.
1. It’s diverse: Real-world data is collected in the context of routine healthcare delivery, which means it reflects the diverse patient populations and real-world treatment patterns encountered in everyday clinical practice. Real-world data can provide a broader and more representative view of patient outcomes and treatment effects.
2. It’s large in size: Real-world data is typically collected from large patient populations, often spanning multiple healthcare systems or databases. This large sample size allows for the detection of rare events, subgroup analyses, and evaluation of treatment effects in specific patient populations that may not be feasible in clinical trials due to limited sample sizes or that collect from point solutions.
3. It’s longitudinal data: Real-world data is often collected over an extended period, allowing for the assessment of long-term outcomes and treatment effects. Clinical trials, on the other hand, are usually conducted over a limited timeframe and may not capture long-term effects or rare events that occur after the trial period.
4. It’s cost and time efficient. Conducting clinical trials can be expensive, time-consuming, and resource-intensive. Real-world data, on the other hand, is already collected as part of routine clinical practice, reducing the need for additional data collection efforts. This makes real-world data a more cost-effective and efficient option for studying treatment outcomes for value-based care models.
Behavioral healthcare needs more conversation within the scientific community to avoid building a value-based healthcare system on rigid standards with small data from point solutions that may not perform well when real practitioners simply wish to practice using their best professional judgement – and innovative to move behavioral health forward.
Will shedding light on the challenges of standardization and outcomes reproducibility help us move behavioral healthcare forward to value-based care?
We’ll be watching.
And, in the meantime we'll be listening. See you next time. Don't forget to subscribe.
Other Blogs
The Plan No One Sees Coming—But Soon Will
Exclusive
Mental Health
Addiction
Drugs
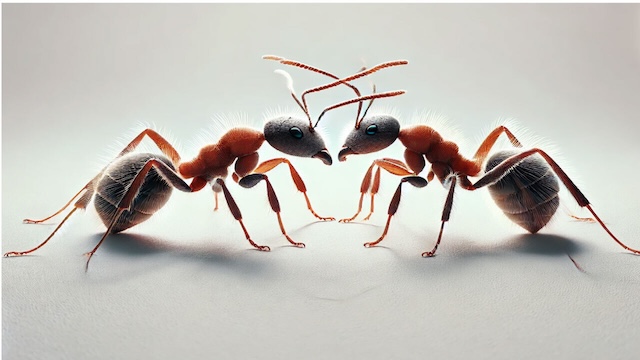
Ant Pheromone Study May Improve Mental Health Outcomes
Exclusive
Mental Health
Addiction
Drugs
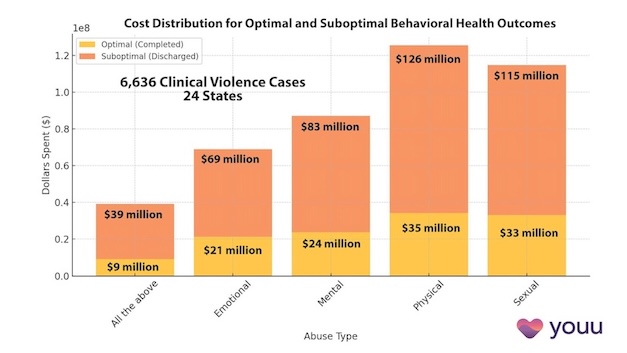
Interrupting Violence Should Be Irresistibly Investable
Exclusive
Mental Health
Addiction
Drugs
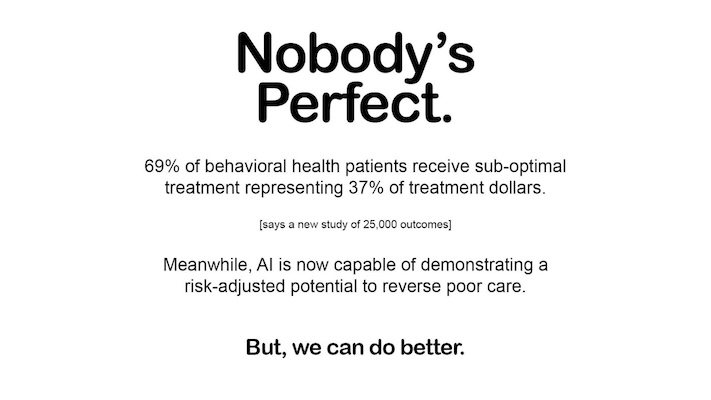
69% of Behavioral Health Patients Receive Sub-Optimal Care According to a New Analysis
Exclusive
Mental Health
Addiction
Drugs
Other Blogs
Have Questions? Lets Meet
Select a time you like to meet with us