Published Date:
Jun 26, 2023
Exclusive
Behavioral Health
AI
Should You Trust AI With Your Healthcare?
Forget out-of-the-box GPT. Here are five interpretability standards for training your AI to recognize human intent.
I was asked this week by the folks at MIT to posit an opinion about whether AI should be trusted in healthcare. And, if so, how would we know if it could be trusted with such a critical application? Of course, the buzz of GPT came to mind and to that, I fear AI in healthcare isn’t as simple as asking GPT a question with your personal browser.
Here’s an analogy. I don’t own an airplane, nor have I ever considered trying to fly one. If I did, I could have a very negative outcome. The same is true with AI and healthcare. Making AI work is a really specialized field if you need it to get off the ground and keep it flying. I’ve spent two decades working with it and I still learn something with every new model.
The following is a real-life application of an AI machine learning model our engineers over at the Youu Health team built and how they used five interpretability standards we feel are important with every design; building trust in the system, verifying before deciding; diagnostic and/or forensic analysis; counterfactual reasoning, and discover new medical insights.
Number one. Build trust. Pick up any trade journal and there is a robust discussion on AI and trust. So, in my examples we’ll hold that trust is the core measure of whether users of AI in healthcare will dare to adopt it – and how our interpretability standards outlined below will encourage it.
Here’s an example
Patient matching and optimization at patient admission is a challenge with Behavioral Healthcare (specifically, substance use and mental health). AI machine learning can help care providers design a personal care journey that increases the probability a patient might avoid a chronic escalation of their original symptoms - long term. Because substance use and/or mental health is a chronic disease, providers often struggle with poor results after the initial acute care cycle. Machine learning promises to increase the probability of success by predicting which patient attributes should be considered a priority when first diagnosing care at admissions. Once your AI model is trained the AI Language Model can describe it.
Diagnostic skills and professional training among providers vary significantly. That’s an ongoing issue with providers. By training a model to order patient attributes for outcome probability, providers can design programs that could increase abstinence over time. As a baseline, abstinence currently averages ~14% at day 360 – an admittedly poor showing by any standard of health care.
Patients often have a broad array of co-morbidity conditions making it time-consuming to determine the risk profile of a patient. Matching that patient to an appropriate treatment program raises the likelihood that patient will have a positive outcome. For example, some patient pathways must first clear primary care. Physiological health issues like detoxification protocols, or care to stabilize diabetes is a requirement before underlying psychological issues are addressed. But, to what degree does this affect the eventual outcome?
Some patients may perform well with out-patient care; others with intensive out-patient and yet others with residential acute care. Often, the amount of standardized data collected data involves about 400 diagnostic examination areas across a broad array of lifestyle and population health characteristics. This is cumbersome and time-consuming making diagnosis difficult because of the nature of documenting that diagnosis and deciding how to get paid for it. The data is simply too large and too complicated for a human to wholly consider, so payment often drives programming. Thus, providers tend to place everyone into the same programs and deal with care variations along the way.
It's clear that some data isn’t meaningful if predicting an inevitable outcome at admissions is the provider’s goal for care. When viewed forensically, the patient’s diagnosis of a care pathway is often complicated with issues masking root causation. Placing the patient into the wrong level of care exacerbates the recovery process and increases cost.
Real-world solution
In a real-life example, performed by our team examining data from 12,500 patients, forensic and counterfactual analysis was used to reduce the number of data considered from 400 to about 20 that showed high correlation to a positive outcome (defined as abstinence from substance use and discharge status after one year post care). Surprisingly, the machine learning model discovered data elements demonstrating the highest probabilities were often those clinicians had never considered. This juxtaposition significantly changed the pathway for many patients.
For example, patients often hide sexual abuse because of stigmatization. So, if a male has been abused by another male, then placing them into a male group therapy session often slows the discovery of the core issue that led to the use of substances he used to block the trauma. In this case, our machine learning model focused on sentiment (positive and negative affect) and modeled an association of that sentiment to twelve potential areas that could be stimulating an observable psychological affect.
Thus, we found it to be very important to verify before deciding which treatment program best matches the highest probability for a successful outcome – while balancing the cost of care with the patient’s viewpoint on what success might look like when completing their care. That tended to increase the value for the patient, the provider and the payer.
In this case, the model proved to produce good results when matching the patient’s attributes to the program’s demonstrated milestones compared to other populations of similar patients. Verification, in this example, required a deep examination of the dataset commonly collected during intake. Since this dataset has common attributes, it isn’t difficult to track how the model works using a forensic examination of this data so humans can understand which patients have better recovery outcomes compared with others grouped by similar bio/psycho/social makeup. Most importantly, we had to show how the model worked to our human counterparts so we created a visualization graph that ordered the associations.
In this case, counterfactual reasoning presented some interesting new discoveries to modify customary treatment giving practitioners a means to exercise creativity to experiment with approaches that could yield new insights with care models. Institutionalizing a counterfactual workflow for training the AI model will improve care, reduce costs, improve outcomes and help healthcare workforces make their work more meaningful and enjoyable thus ushering in an era of personalize care for our patients.
Other Blogs
The Plan No One Sees Coming—But Soon Will
Exclusive
Mental Health
Addiction
Drugs
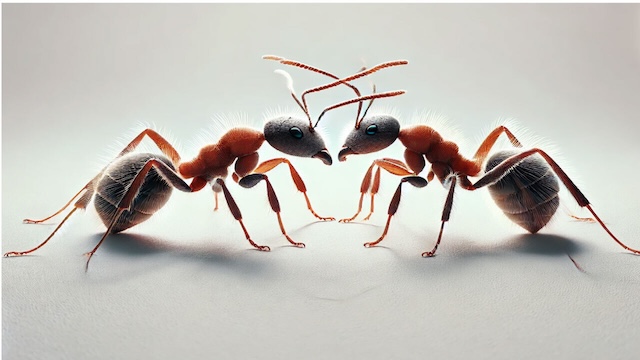
Ant Pheromone Study May Improve Mental Health Outcomes
Exclusive
Mental Health
Addiction
Drugs
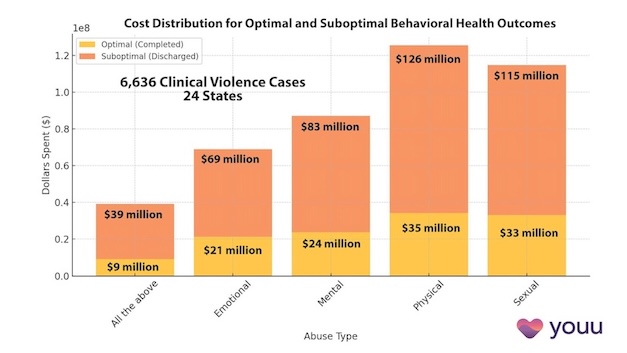
Interrupting Violence Should Be Irresistibly Investable
Exclusive
Mental Health
Addiction
Drugs
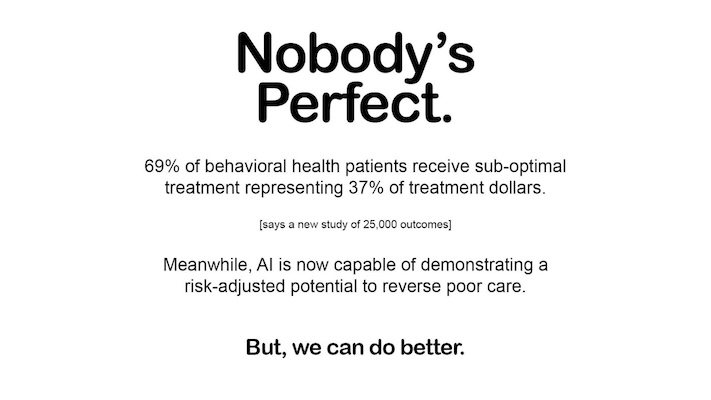
69% of Behavioral Health Patients Receive Sub-Optimal Care According to a New Analysis
Exclusive
Mental Health
Addiction
Drugs
Other Blogs
Have Questions? Lets Meet
Select a time you like to meet with us